In a recent blog, we articulated some of the ways that AI Governance is a unique and complicated function in the enterprise. The top line is that AI governance performs a “control center” function that requires integration with an incredibly broad range of tools and processes within the enterprise. Due to the breadth of different use cases, the variety of AI systems, the emerging compliance landscape, and the emergence of a vibrant 3rd-party model landscape, AI governance has a broader mandate than previous narrow governance approaches (e.g., privacy and data) or technical robustness checks.
AI governance sits atop these other functions, aggregating and distilling all information about an AI system to enable more responsible decision making about AI’s use at the enterprise.
So now that we’re on the same page about AI governance’s “control center” function, let’s dive into the other aspects of the responsible AI (RAI) stack!
This blog will highlight a simple schematic of an “ideal responsible AI stack” that interfaces with your AI governance platform.
This stack will allow you to both measure what’s going on in your system and manage how it is developed and deployed. Along the way we’ll describe these functions as well as some companies that represent them well. As there are a number of different tools, it’s critical that an AI governance platform serves as an orchestration layer to ensure efficiency and effective application of the stack.
The Responsible AI Stack: An Overview
To effectively govern AI systems, organizations need a comprehensive set of tools and processes that span the entire AI lifecycle, what we’ll call the "Responsible AI Stack.” The stack is divided into three main sections: tools for Measuring AI Systems, which provide the data and insights needed for effective governance; tools for “Managing” AI Systems, which control and influence the AI system itself; and dual-purpose tools.
It’s important to point out that each section here is complicated.
Bringing AI Governance into your organization doesn’t simplify the individual challenge of any of these functions.
- Trust & Safety teams will still be needed to set up the best guardrails, monitor systems for issues, and respond accordingly.
- Privacy teams will need to carefully manage user data.
- Prompt engineers and ML teams will need to focus on building performant models, and the list goes on!
So while AI Governance helps orchestrate these functions and enables AI development to become a more seamless multi-stakeholder endeavor, each area is a function that needs to be pursued with focus and specialized expertise.
The burgeoning Ops ecosystem is a testament to this complexity, as not every enterprise has in-house expertise for every function, and thus may be better off procuring the right tool. As we go through and describe these different sections we will list a few examples of companies that represent this function. It’s important to note that some companies don’t provide functionality that exists in one category. We focus on highlighting companies that have a relatively circumscribed “lane”. Larger companies like Microsoft, DataBricks, Cloudflare, Google or Amazon provide services in many areas, that could include model hosting and inference services, AI security, databases, etc.
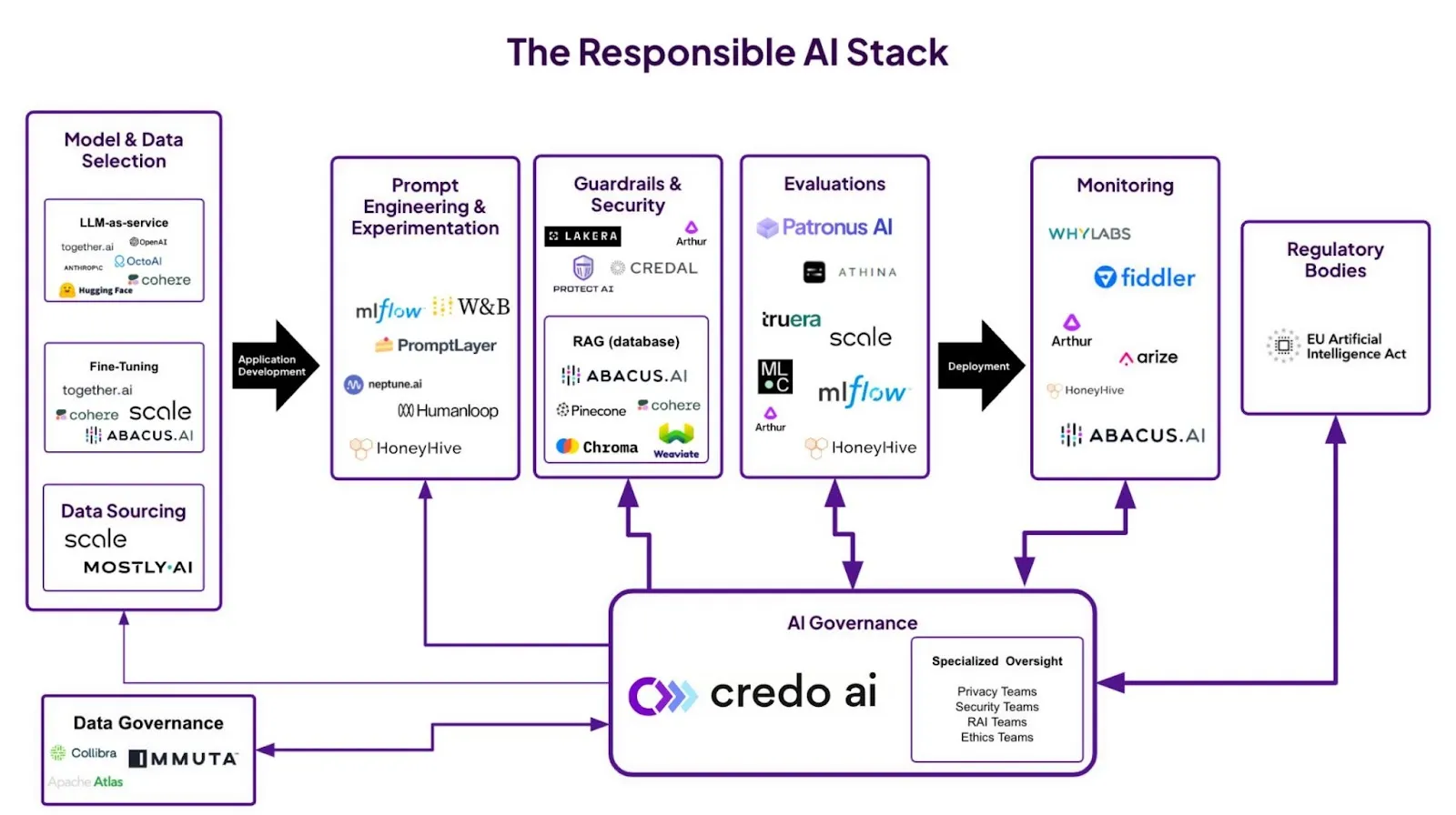
Responsible AI Stack: This schematic displays the overall responsible AI stack (with a focus on generative AI applications) and their relationship to AI Governance. The thickness of the line indicates the relationship strength between AI Governance and that function. As the AI system moves from initial conception to production (moving left to right) governance becomes increasingly critical. Ideally, governance is impacting the system from the beginning, which will ensure alignment and compliance later in the process.
Measuring AI Systems
Measuring AI involves tools and processes that provide transparency, visibility, and insights into the performance, behavior, and impact of AI models. These components help organizations understand what their AI systems are doing, how well they're doing it, and what risks or issues may need to be addressed. Key categories of measuring tools include:
Monitoring / Observability - AI monitoring (or “observability”) platforms provide real-time visibility into the performance, behavior, and impact of AI models in production environments. These tools track key metrics like accuracy, latency, and resource utilization, as well as monitoring for anomalies, drift, and potential biases. Traditionally, AI monitoring has been the responsibility of ML engineering and operations teams, who use these tools to ensure models are performing as expected and to troubleshoot issues. However, as AI systems become more complex and consequential, monitoring is also becoming a critical tool for AI governance. This relationship becomes even more important as organizations also monitor the usage of AI systems by their employees, tracking the kinds of prompts and tasks that general-purpose AI systems are supporting. By integrating with AI governance platforms, monitoring tools can provide governance teams with real-time insights into model behavior and use, flagging potential risks or compliance issues for immediate action. This integration enables proactive governance, allowing organizations to identify and mitigate risks before they result in harm.
- Example companies building monitoring tools include Fiddler, WhyLabs, Arthur, Arize, Galileo and HoneyHive.
Evaluations - Evaluation tools and platforms help organizations assess the performance, fairness, robustness, transparency, and other key characteristics of AI models. These tools often include metrics, frameworks, and methodologies for testing models against various criteria, such as accuracy, bias, explainability, and safety. Traditionally, model evaluation has been primarily a technical function, performed by data science and ML engineering teams as part of the model development and validation process. However, as the need for responsible and transparent AI grows, model evaluation is becoming a critical component of AI governance. Evaluation tools can integrate with AI governance platforms to provide standardized, auditable assessments of model performance and risks, informing governance decisions around model approval, monitoring, and control. This integration helps ensure that models are thoroughly vetted before deployment and continuously evaluated once in production.
A special-class of evaluation support are benchmarks. While not a specific tool, these standardized datasets give a perspective on model performance that is comparable across model providers. Many organizations build benchmarks, including industry groups, nonprofits like the MLCommons, and many academic labs.
- Key tool providers in this space are Apollo, Weights & Biases, Patronus AI, Athina, Truera, Scale, Galileo and Arthur. Nonprofits like MLCommons and METR are also relevant here.
Managing AI Systems
Managing AI systems encompasses tools and processes that allow organizations to control, guide, and oversee the development and deployment of AI models. These components help ensure that AI systems are built and used responsibly, ethically, and in compliance with relevant laws and standards. Key categories of management tools include:
Model selection - Model selection tools help organizations choose the most appropriate AI models and datasets for their specific use cases. These platforms may focus on proprietary models or may be a repository and broker of open-source models. OpenAI, Anthropic, Cohere, and Google, while Hugging Face, Together.AI and OctoAI are examples of the latter. Some of these platforms may also include fine-tuning services (see the next section) to further customize the model. This function has become prominent with the advent of genAI, but existed previously when AI systems were built atop off-the-shelf models for common tasks (e.g., word embeddings or facial recognition systems).
Traditionally, model selection has been the domain of data science and ML engineering teams, who are responsible for identifying the right models to meet business requirements. However, as AI governance becomes a more pressing concern, these teams need to work closely with governance functions to ensure that selected models and data align with organizational policies, ethical principles, and legal requirements. Model and data selection tools can integrate with AI governance platforms to provide transparency into the provenance, characteristics, and potential risks of chosen models and datasets, enabling informed governance decisions.
- There are many model providers, whose models differ in their degree of openness. Generally closed models are provided by OpenAI, Anthropic, Google, & Cohere, while generally open models are built by a number of entities including Meta and Mistral, nonprofits like Cohere for AI. Access to these models, in terms of fine-tuning and inference services can additionally be provided by platforms like HuggingFace, OctoML, and TogetherAI.
Data Sourcing - Data sourcing platforms and processes are used to acquire the data needed to train, test, and validate AI models. These tools help organizations find and ingest diverse, representative, and high-quality data from various sources, including internal databases, public datasets, and third-party providers. Data can be sourced from open datasets like Common Crawl and LAION, or from providers that help create custom datasets, either through human labor or synthetic data creation. Data sourcing tools can integrate with AI governance platforms to provide transparency into the provenance, quality, and potential biases of sourced data, enabling governance teams to assess data-related risks and ensure compliance with data usage policies. An even simpler integration is for Data Governance teams to take over responsibility for data sourcing, and pass that information directly to AI Governance platforms. Either way, governing data sources helps ensure that AI models are built on a foundation of reliable, representative, and ethically sourced data.
- Data sourcing is a complicated pipeline and it depends on what your purpose is. There are open datasets like CommonCrawl and LAION, which are used as part of foundation model training, and providers that help create new data like Scale AI and mostly.AI.
Fine-tuning and RAG (Retrieval Augmented Generation) - Tools and techniques for adapting base models to specific use cases and enhancing their performance by incorporating external knowledge. Fine-tuning involves training base models on additional data to improve their accuracy or specialize them for particular tasks. RAG systems, such as those using vector databases, enable models to retrieve and incorporate relevant information from external databases or knowledge bases during the generation process. These approaches help organizations build more accurate, context-aware, and controllable AI systems on top of general-purpose base models.
- Fine-tuning is provided by a subset of model providers.
- Vector databases are provided by tools like Pinecone, Weaviate, and Chroma.
Prompt Engineering & Experimentation Tracking - Prompt engineering and experimentation tools enable organizations to design effective prompts for AI models and test them in a controlled environment. These tools help teams understand how different prompts can influence model behavior and output, allowing them to optimize prompts for specific use cases while mitigating potential risks. Traditionally, prompt engineering has been an iterative process performed by data scientists and ML engineers as they develop and refine AI applications. However, as the impact of prompts on model behavior becomes clearer, prompt engineering is increasingly a focus for AI governance teams. By integrating with AI governance platforms, prompt engineering tools can help governance teams assess the risks and ethical implications of different prompts, provide guidance on prompt best practices, and monitor prompt usage in production to ensure compliance with policies.
- Examples include MLflow, Weights & Biases, Galileo, PromptLayer, Neptune.ai, Humanloop, and HoneyHive.
Guardrails & Security - Guardrails and security tools help organizations implement safety controls, content filters, and other mechanisms to prevent unintended or harmful model behaviors, as well as protect against cyber threats and adversarial attacks. These tools have become increasingly important in the genAI era, as the open-ended nature of large language models and their ability to generate diverse content types (e.g., text or images) can lead to potential risks and vulnerabilities.
Guardrail tools enable organizations to set boundaries and constraints on model outputs, filter out inappropriate or offensive content, and ensure models operate within predefined safety limits. Security tools, on the other hand, focus on protecting AI systems from external threats, such as data poisoning, model inversion, or adversarial examples, which can compromise model integrity and lead to unintended behaviors. Providers offer solutions to detect and defend against these threats. By combining guardrails and security measures, organizations can create a robust defense system to mitigate risks and ensure the safe and responsible deployment of AI systems.
- Examples of guardrails include Arthur AI and Guardrails.AI.
- Security tools include Lakera, HiddenLayer, and Robust Intelligence.
Orchestration and Oversight
Some components of the Responsible AI Stack serve dual roles, supporting both measurement and management functions, which we describe as “orchestration and oversight”. These foundational layers provide the data, governance, and oversight mechanisms that enable effective AI governance across the lifecycle. Key systems include:
Data Governance - Data governance frameworks and tools help organizations manage the availability, usability, integrity, and security of the data used for AI. This includes processes and technologies for data cataloging, lineage tracking, quality assurance, access control, and compliance with data privacy regulations. Key players in the data governance space include Collibra, Immuta, and Atlas. Traditionally, data governance has been a function of IT and data management teams, who are responsible for ensuring that data is accurate, consistent, and secure across the organization. However, with the rise of AI and the increasing importance of data in AI systems, data governance is becoming a critical component of AI governance. Data governance tools can integrate with AI governance platforms to provide a centralized view of data assets, policies, and risks, enabling governance teams to make informed decisions about data usage in AI projects. This integration ensures that AI systems are built on a foundation of well-governed, trustworthy data and that data-related risks are effectively managed throughout the AI lifecycle.
- Key players include Collibra, Immuta, and Atlas.
Specialized Oversight Functions - Specialty oversight functions, such as privacy, security, and ethics teams, play a critical role in ensuring that AI systems are developed and deployed responsibly. These teams bring specific expertise and perspectives to the governance process, helping to identify and mitigate risks related to their respective domains. For example:
- Privacy teams ensure that AI systems comply with data privacy regulations and protect individual rights to privacy and data protection.
- Security teams assess the security risks associated with AI systems and implement controls to protect against cyber threats, data breaches, and adversarial attacks.
- Ethics teams provide guidance on the ethical implications of AI systems, helping to ensure that AI is developed and used in ways that are fair, transparent, and aligned with organizational values.
Traditionally, these oversight functions have operated somewhat independently, focusing on their specific areas of concern. However, as AI systems become more complex and pervasive, effective AI governance requires close collaboration and integration between these specialties. AI governance platforms can provide a centralized hub for these oversight functions, enabling them to share knowledge, coordinate activities, and provide integrated guidance and risk assessments. This collaboration helps ensure that AI governance is holistic and multidisciplinary, taking into account the full range of risks and considerations associated with AI systems.
- Certain subsets of these functions may have tools that support them. For instance, Vanta and Drata can help with some forms of security compliance, while OneTrust can support GDPR compliance. However, the actual functions (e.g., Privacy protection) generally need to be supported by expert, empowered teams who can represent and enforce their mandate through the organization.
AI Governance - AI Governance platforms, such as Credo AI, sit at the top of the Responsible AI Stack, providing the overarching governance layer that integrates and orchestrates the various measuring and managing tools to enable comprehensive, end-to-end governance of AI systems. Beyond their integrative function, AI Governance platforms also enable measuring AI system risk and compliance, managing policies, and enabling auditable human oversight. They provide a centralized dashboard for monitoring the performance, risks, and compliance of AI systems across the organization, as well as tools for defining and enforcing governance policies and workflows.
AI Governance platforms work closely with specialized oversight functions, such as privacy, security, and ethics teams, to ensure that AI systems are developed and deployed in accordance with organizational values, industry best practices, and regulatory requirements. They also provide a framework for documenting and auditing AI governance processes, enabling organizations to demonstrate compliance and build trust with stakeholders. As AI systems become more complex and widely adopted, AI Governance platforms will play an increasingly critical role in helping organizations navigate the challenges and opportunities of AI responsibly and ethically.
- A great example of an AI Governance platform is Credo AI of course! What other example do you think we’d pick?
Conclusion

As the AI landscape continues to evolve at a rapid pace, with the rise of generative AI and the increasing adoption of AI across industries, the need for effective AI governance has never been more pressing. The Responsible AI Stack provides a comprehensive framework for managing the risks and opportunities associated with AI, enabling organizations to develop and deploy AI systems in a safe, ethical, and compliant manner.
However, it's important to recognize that AI governance is not a one-size-fits-all solution. Each component of the Responsible AI Stack - from data sourcing and model selection to monitoring and specialized oversight functions - requires dedicated expertise and focus. Effective AI governance is a collaborative effort that involves multiple stakeholders across the organization, including data scientists, ML engineers, privacy experts, security teams, and ethics committees.
This is where AI Governance platforms like Credo AI come in. By providing a centralized hub for integrating and orchestrating the various tools and processes involved in responsible AI development, Credo AI enables organizations to build a cohesive and effective AI governance framework. Credo AI's platform empowers teams to work together seamlessly, sharing knowledge, coordinating activities, and providing integrated risk assessments and guidance.
But Credo AI is not a replacement for the specialized expertise and tools required for each component of the Responsible AI Stack. Rather, it is a critical enabler that helps organizations bring together the best of breed tools and talent to create a robust and comprehensive approach to AI governance.
As we look to the future, it's clear that AI will continue to transform industries and society in profound ways. By investing in the Responsible AI Stack and partnering with AI Governance platforms like Credo AI, organizations can position themselves to harness the power of AI while mitigating its risks and challenges. The path to responsible AI is not always easy, but with the right tools, processes, and collaboration, it is a journey that we can all navigate together.
DISCLAIMER. The information we provide here is for informational purposes only and is not intended in any way to represent legal advice or a legal opinion that you can rely on. It is your sole responsibility to consult an attorney to resolve any legal issues related to this information.